Uncovering the Key Differences between Data Lakehouse and Data Warehouse: A Guide for Business Owners
- Swapnil
- Dec 15, 2024
- 4 min read
Updated: Dec 16, 2024
In today's data-driven world, businesses are constantly looking for effective ways to manage and analyze vast amounts of data. The emergence of big data technologies has led to two main solutions: Data Lakehouse and Data Warehouse. Understanding the differences between these solutions is essential for business owners who want to optimize their data strategies and remain competitive.
Understanding Data Warehouses
Historically, data warehouses have been the go-to solution for businesses that need to store and retrieve large quantities of structured data. A data warehouse organizes data into tables, allowing for complex SQL queries. This structured approach is ideal for business intelligence, analytics reporting, and analyzing historical data.
Typically, data warehouses operate using the Extract, Transform, Load (ETL) framework. This means that data must be processed and transformed into a clean, consistent format before loading it into the warehouse. This guarantees that the data is high-quality and ready for analysis.
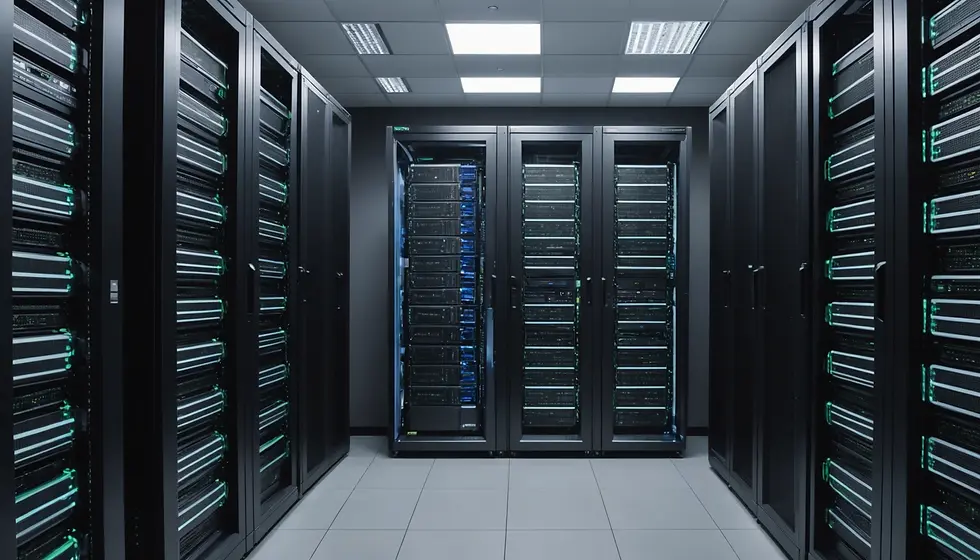
The structured nature of data warehouses makes them perfect for organizations that prioritize data quality. According to a report by Gartner, companies that maintain a strong data quality framework see 30% or more improvement in decision-making effectiveness.
However, this structure means they struggle to handle diverse unstructured data types, such as text or images. For example, a business using social media feedback to improve products might find a data warehouse limiting as it cannot adequately store and analyze text comments and user images.
Exploring Data Lakehouses
The Data Lakehouse architecture has emerged as a next-generation solution that combines the best features of both data lakes and data warehouses. A data lakehouse supports the storage of structured and unstructured data in a single repository, allowing businesses to perform SQL analytics while enjoying the flexibility of data lakes.
Data lakehouses utilize an open data format, fostering extensive analytical capabilities, including machine learning and real-time processing. This architecture can accommodate the rapid influx of data from sources like IoT devices and social media, facilitating insights across various data types.
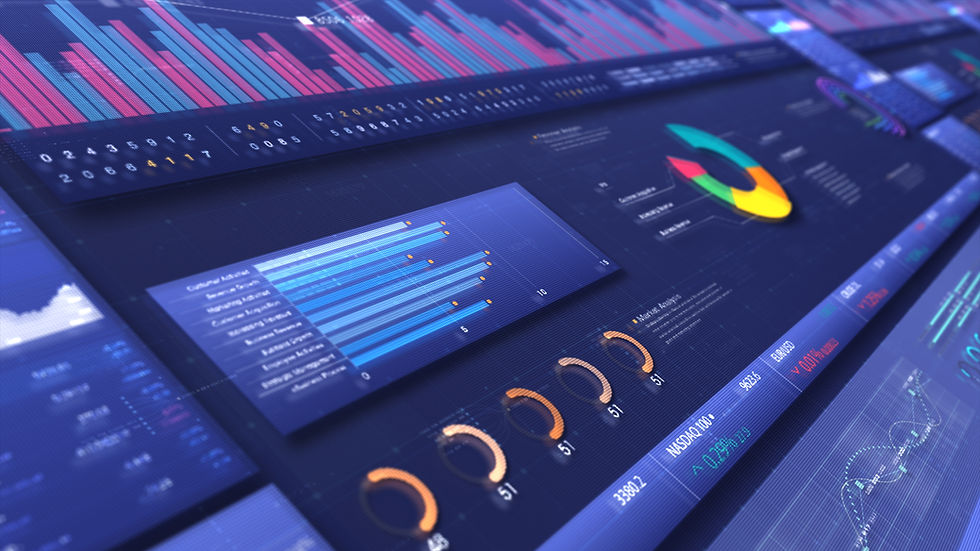
With a data lakehouse, organizations benefit from a schema-on-read approach. This means data can be ingested in its raw form, providing greater flexibility. For instance, firms can analyze clickstream data from websites without preprocessing it beforehand.
Key Differences
When choosing the best solution for your business, consider several key factors:
1. Data Structure
Data warehouses primarily store structured data. They excel in ensuring data quality, consistency, and reliability. For example, a retail business might rely on a data warehouse to track sales and inventory levels effectively. In contrast, data lakehouses accommodate both structured and unstructured data, making them a suitable option for companies dealing with diverse data types, such as multimedia content and logs.
2. Data Processing
Data warehouses utilize an ETL process, which requires cleaning and transforming data before storage. For example, a bank may take days to prepare financial reports due to this lengthy process. On the other hand, data lakehouses allow for a schema-on-read approach—data can be stored in its original form for immediate analysis, speeding up the data ingestion process.
3. Analytics
While traditional data warehouses are great for batched analytics and historical reporting, data lakehouses support a wider variety of analytics capabilities. These include real-time analytics and advanced machine learning applications. For example, streaming data from customer transactions can be analyzed immediately in a data lakehouse to detect fraudulent activity while data warehouses may only analyze these transactions at a later date.
4. Cost
Data warehouses can be costly due to storage and processing expenses, especially when scaling operations. According to a study by Forrester, companies can save up to 60% on storage costs by utilizing data lakehouses. With their ability to use cost-effective cloud storage options, lakehouses provide an affordable alternative, especially for businesses expecting data growth.
5. Use Cases
The best solution often depends on the specific needs of your business. Data warehouses are suited for structured reporting and dashboard creation, often found in industries like finance, healthcare, and retail, where data integrity is vital. Conversely, data lakehouses excel in exploratory data analysis, real-time analytics, and machine learning projects, making them ideal for organizations in sectors like technology and telecommunications.
Choosing the Right Solution
To decide between a data lakehouse and a data warehouse, consider your organization’s specific requirements. If your business relies heavily on structured data, needs high-quality analytics reporting, and prioritizes data governance, a data warehouse might be the better choice. For instance, a healthcare organization focusing on patient records might benefit from the structured and compliant nature of a data warehouse.
In contrast, if your business thrives on dynamic and varied data analysis and requires agility in data processing, a data lakehouse may be more beneficial. Industries like technology that prioritize innovation often find the adaptability of the lakehouse to be particularly advantageous.
Future Trends in Data Management
The data management landscape is continuously evolving, and both data warehouses and data lakehouses are being refined to meet new business challenges. Hybrid solutions that harness the strengths of both models are becoming increasingly common, providing businesses with better flexibility.
Investments in AI and machine learning technologies will shape future data strategies. As more organizations leverage data for a competitive edge, the functionalities of both data lakehouses and warehouses will expand, offering businesses even greater capabilities in data analysis.
Final Thoughts
Choosing between a data lakehouse and a data warehouse is an important decision that can significantly affect your business's data management strategy. By grasping the key differences, advantages, and potential use cases for each solution, business owners can make informed decisions that align with their specific goals and requirements.
Ultimately, the right choice will depend on your data needs and the complexity of your analytics objectives. The future of data management is promising, and with the right approach, organizations can unlock significant value from their data assets.
Investing in either a data warehouse or a data lakehouse positions businesses for successful data-driven decision-making and innovation, ensuring they thrive in an increasingly data-focused landscape.
Comments